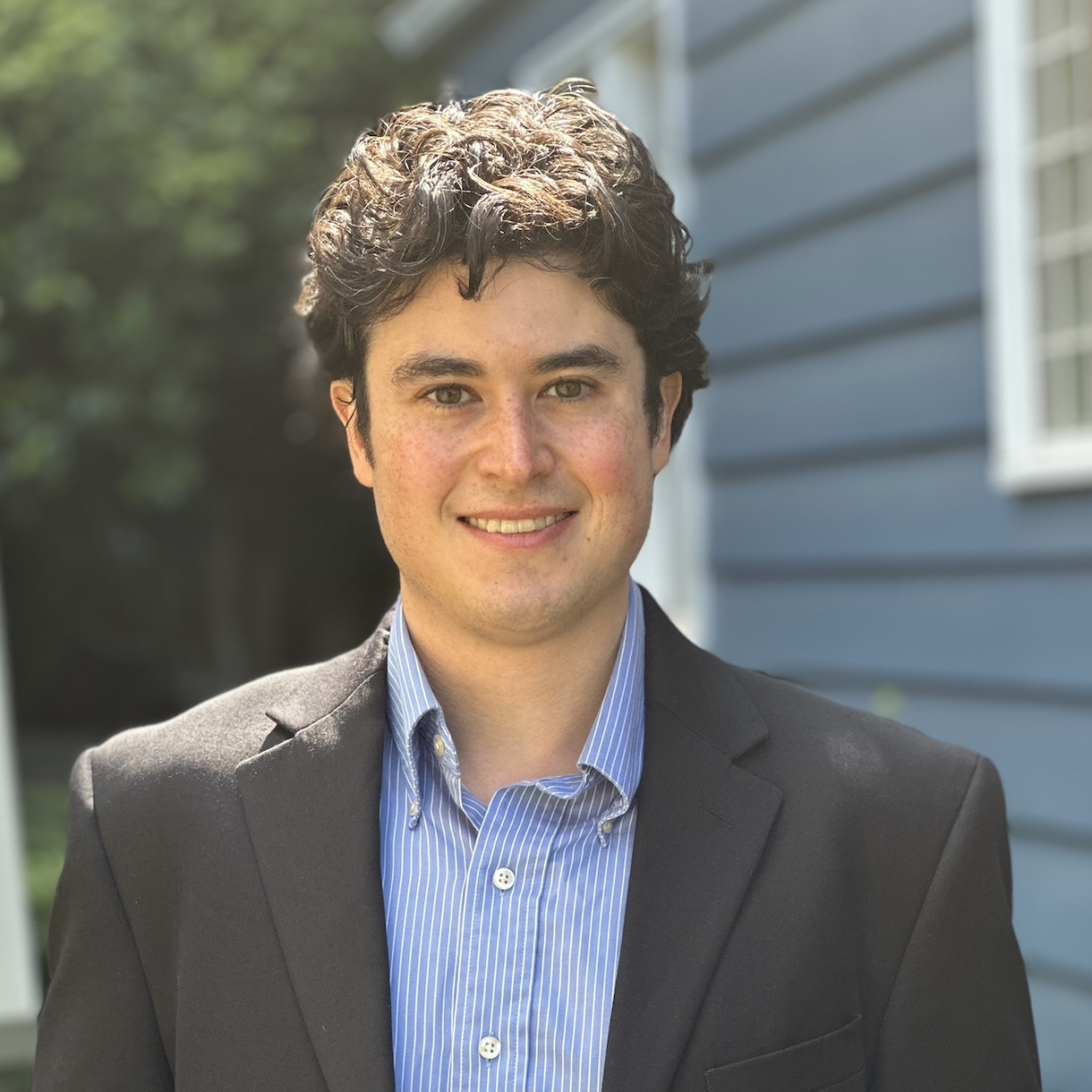
Sam Otto
Biography
Dr. Otto joined the Cornell MAE faculty as an Assistant Professor in July 2024. Prior to this, he was a Postdoctoral Scholar at the AI Institute in Dynamic Systems at the University of Washington. He received his Ph.D in mechanical and aerospace engineering from Princeton University in May 2022 and his B.S. in aeronautics and astronautics from Purdue University in 2016. He enjoys science fiction, fantasy, and weightlifting and can usually be found in a coffee shop with a math textbook.
Research Interests
Recent advances in machine learning and artificial intelligence yield promising tools to efficiently model, forecast, and control high-dimensional nonlinear systems such as fluid flows using data collected from experiments and high-fidelity simulations. However, the reliability of data-driven models must be established before they can be used in most engineering applications. Dr. Otto’s research focuses on developing principled scientific machine learning algorithms and comprehensive theory explaining their capabilities and fundamental limitations in continuum mechanics applications. This includes developing algorithms to accelerate forecasts either by constructing simplified reduced-order models, or by accelerating numerical solvers using learned solution operators for preconditioning. On the theoretical side, a major goal is to understand what can and can’t be learned from limited data, and what additional physical information such as symmetry, smoothness, or scale hierarchy can be leveraged to enable data-efficient learning.
Selected Publications
- N. Boulle, D. Halikias, S. E. Otto, and A. Townsend, 2024, Operator learning without the adjoint, arXiv:2401.17739
- S. E. Otto, N. Zolman, J. N. Kutz, and S. L. Brunton, 2023, A unified framework to enforce, discover, and promote symmetry in machine learning, arXiv:2311.00212
- S. E. Otto, G. R. Macchio, and C. W. Rowley, 2023, Learning nonlinear projections for reduced-order modeling of dynamical systems using constrained autoencoders, Chaos, Vol. 33, Iss. 11
- S. E. Otto, A. Padovan, and C. W. Rowley, 2023, Model reduction for nonlinear systems by balanced truncation of state and gradient covariance, SIAM Journal on Scientific Computing, Vol. 45, Iss. 5
- S. E. Otto and C. W. Rowley, 2022, Inadequacy of linear methods for minimal sensor placement and feature selection in nonlinear systems: a new approach using secants, Journal of Nonlinear Science, Vol. 32, No. 69